Data Cleaning: Types of Missing Values (and How to Handle Them)
Data cleaning happens early in the data analysis process and is a critical aspect of data analytics. Simply put, data cleaning is the process of preparing and validating data—usually before your core analysis.
Data cleaning is important because it helps to ensure (and improve) the quality of your data, and as a result, impacts the quality of any analysis based on that data. As so many in the field will tell you: garbage in, garbage out. The rest of your process could be absolutely perfect but your data will still need cleaning!
While most of the work good data cleaning requires is in detecting and correcting “rogue data” (incomplete, inaccurate, irrelevant, corrupt or incorrectly formatted data), it’s also important to deal with missing data. here are three common ways to do this:
- You can remove entries associated with the missing data
- You can impute (or guess) the missing data, based on other, similar data
- Or you can simply flag the data as “missing” or “0” (depending on whether you’re working with qualitative or quantitative data)
But be careful! It’s important to note that removing or guessing the missing values can lead to missing other information or erroneously reinforcing existing patterns in the data. So it’s best to exercise these methods with some awareness and caution.
Featured presenters
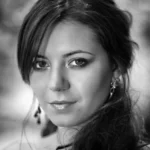
Dana Daskalova
Course Reviewer & Writer, CareerFoundry Data Analytics Tutor
Dana Daskalova started her career as a data scientist from scratch. Initially a humanities alumnus, she embraced statistics and mathematics during her studies at the University of Vienna, and began tutoring others in the field. After graduating in Vienna, where she also worked as a freelance research analyst, she joined a management consulting agency in London, got acquainted with behavioural science, and started applying statistical modelling to predict customer behaviour for various retail and tech giants. Later on, Dana acquired in-depth knowledge with risk assessment and credit scoring working as a data modeller for Experian. Something was missing, though! Destiny brought her to CareerFoundry, where she’s written and reviewed courses for the Data Analytics Program, and tutors aspiring data analysts. All of these experiences have made teaching and helping others a real passion for Dana. In her free time, she loves street photography and digging into medical data and research.